Search Constraints
Filtering by:
Discipline
Other
Remove constraint Discipline: Other
Discipline
Science
Remove constraint Discipline: Science
1 - 4 of 4
Number of results to display per page
View results as:
Search Results
- Creator:
- Ruas, Terry, Ferreira, Charles H. P., Grosky, William, França, Fabrício O., and Medeiros, Débora M. R,
- Description:
- The relationship between words in a sentence often tell us more about the underlying semantic content of a document than its actual words, individually. Recent publications in the natural language processing arena, more specifically using word embeddings, try to incorporate semantic aspects into their word vector representation by considering the context of words and how they are distributed in a document collection. In this work, we propose two novel algorithms, called Flexible Lexical Chain II and Fixed Lexical Chain II that combine the semantic relations derived from lexical chains, prior knowledge from lexical databases, and the robustness of the distributional hypothesis in word embeddings into a single decoupled system. In short, our approach has three main contributions: (i) unsupervised techniques that fully integrate word embeddings and lexical chains; (ii) a more solid semantic representation that considers the latent relation between words in a document; and (iii) lightweight word embeddings models that can be extended to any natural language task. Knowledge-based systems that use natural language text can benefit from our approach to mitigate ambiguous semantic representations provided by traditional statistical approaches. The proposed techniques are tested against seven word embeddings algorithms using five different machine learning classifiers over six scenarios in the document classification task. Our results show that the integration between lexical chains and word embeddings representations sustain state-of-the-art results, even against more complex systems. Github: https://github.com/truas/LexicalChain_Builder
- Keyword:
- document classification, lexical chains, word embeddings, synset embeddings, chain2vec, and natural language processing
- Citation to related publication:
- Terry Ruas, Charles Henrique Porto Ferreira, William Grosky, Fabrício Olivetti de França, Débora Maria Rossi de Medeiros, "Enhanced word embeddings using multi-semantic representation through lexical chains", Information Sciences, 2020, https://doi.org/10.1016/j.ins.2020.04.048
- Discipline:
- Other, Science, and Engineering
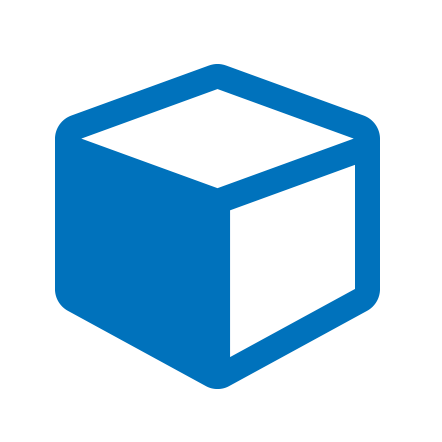
- Creator:
- Richter, Jack and Pettersen, Claire
- Description:
- Radar observations supply detailed information about the structure and evolution of precipitation. These observations allow one to evaluate the macro- and/or micro-physical properties of precipitation at high spatial and temporal resolution. This dataset provides a nearly continuous collection of radar observations from a Metek Micro Rain Radar 2 (MRR) in Marquette, Michigan, USA (MQT). The MRR is a relatively low-cost, low-power K-band (24 GHz) profiling radar that scans the atmosphere at a fixed 90° zenith angle (i.e., directly overhead). The MRR in MQT is configured such that observations are provided every minute at a vertical resolution of 100m up to 3000m AGL (note: due to ground clutter, the effective operating range is 400m–3000m AGL). The MRR data are processed using IMProToo (Maahn and Kollias, 2012; https://doi.org/10.5194/amt-5-2661-2012) to increase the sensitivity of the radar to -10 dBZ and are “de-noised” using a principal component analysis method on the MRR raw power spectra to remove interference from a nearby broadcasting tower (Pettersen et al., 2020; https://doi.org/10.1175/JAMC-D-19-0099.1). Within this dataset, users will find observations such as the equivalent reflectivity factor, Doppler velocity, and reflectivity power spectra.
- Keyword:
- radar, snowfall, precipiation, microphysics, and in situ
- Citation to related publication:
- https://doi.org/10.1175/JAMC-D-19-0099.1, https://doi.org/10.1175/BAMS-D-19-0128.1, and https://doi.org/10.1029/2022JD037132
- Discipline:
- Other and Science
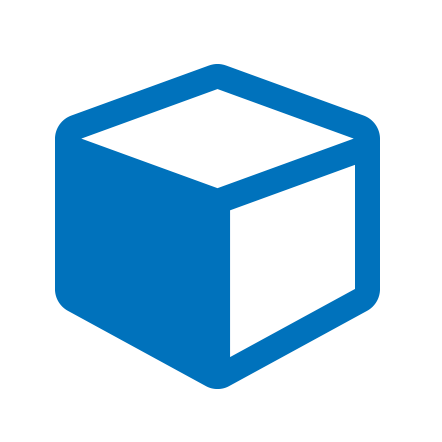
- Creator:
- Lee, Shih Kuang, Tsai, Sun Ting, and Glotzer, Sharon C.
- Description:
- The trajectory data and codes were generated for our work "Classification of complex local environments in systems of particle shapes through shape-symmetry encoded data augmentation" (amidst peer review process). The data sets contain trajectory data in GSD file format for 7 test systems, including cubic structures, two-dimensional and three-dimensional patchy particle shape systems, hexagonal bipyramids with two aspect ratios, and truncated shapes with two degrees of truncation. Besides, the corresponding Python code and Jupyter notebook used to perform data augmentation, MLP classifier training, and MLP classifier testing are included.
- Keyword:
- Machine Learning, Colloids Self-Assembly, Crystallization, and Order Parameter
- Citation to related publication:
- https://doi.org/10.48550/arXiv.2312.11822
- Discipline:
- Other, Science, and Engineering
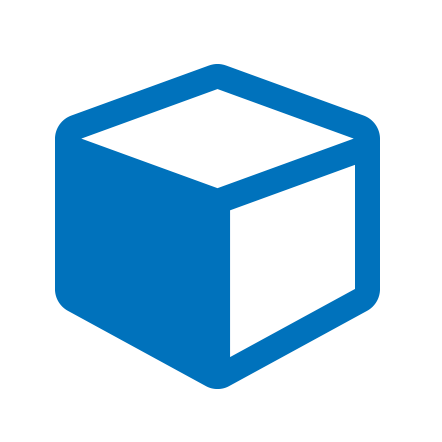
- Creator:
- Mirshams Shahshahani, Payam
- Description:
- Investigating minimum human reaction times is often confounded by the motivation, training, and state of arousal of the subjects. We used the reaction times of athletes competing in the shorter sprint events in the Athletics competitions in recent Olympics (2004-2016) to determine minimum human reaction times because there's little question as to their motivation, training, or state of arousal. The reaction times of sprinters however are only available on the IAAF web page for each individual heat, in each event, at each Olympic. Therefore we compiled all these data into two separate excel sheets which can be used for further analyses.
- Keyword:
- minimum reaction time, sprinter, Olympics, Athletics, sex difference, starting block, and false start
- Citation to related publication:
- Mirshams Shahshahani P, Lipps DB, Galecki AT, Ashton-Miller JA (2018) On the apparent decrease in Olympic sprinter reaction times. PLoS ONE 13(6): e0198633. https://doi.org/10.1371/journal.pone.0198633
- Discipline:
- Engineering, Health Sciences, Science, Other, and General Information Sources