Search Constraints
1 - 9 of 9
Number of results to display per page
View results as:
Search Results
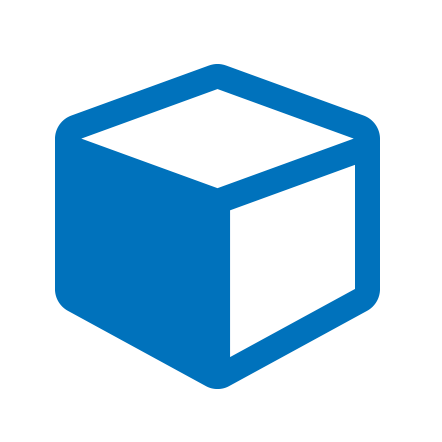
- Creator:
- Quartey, Nii-Boi A and Liemohn, Michael W
- Description:
- This dataset contains results from the BATS-R-US multispecies MHD code simulating the solar wind interaction at Mars at the following orbital and solar cycle locations: solar maximum at perihelion, solar maximum at aphelion, solar minimum at perihelion, solar minimum at aphelion. These simulations contain results with and without the crustal magnetic fields and includes variables such as the magnetic field and ion density. and 2025-02-25: The metadata in this dataset has been updated in response to reviewer comments during the journal review process. Additional Tecplot 360 EX file containing MAVEN multifluid MHD simulation data added. The multifluid MHD simulation result from this result is from the MAVEN simulation library. The simulation result is run for 25,000 iterations.
- Keyword:
- mars, magnetosphere, magnetotail, current sheet, asymmetry, multispecies, multifluid, MHD, and MAVEN
- Discipline:
- Science
2. 12 hour data for magnetospheric simulations for a multifluid plasma for 8 different configurations
- Creator:
- Trung, Huy-Sinh, Liemohn, Michael W, and Ilie, Raluca
- Description:
- The goal of this research was to understand structures where the solar wind plasma contribution to the total plasma was equal to the ionospheric plasma. This simulation was performed over a simulation time of 12 hours for 4 different plasma compositions for 2 different solar wind profiles., The SWMF used the Block Adaptive Tree Solar wind Roe-type Upwind Scheme version 9.20. It can be found at http://csem.engin.umich.edu/tools/swmf/downloads.php. These data can be processed using the simulation code deposited at the Deep Blue Data record indicated in the "Citation to related material" field., and To cite this data set: Trung, H.-S., Liemohn, M., W., Ilie, R. (2019). 12 hour data for magnetospheric simulations for a multifluid plasma for 8 different configurations [Data set]. University of Michigan Deep Blue Data Repository. https://doi.org/10.7302/fwq2-ey41
- Keyword:
- Space Physics, Magnetospheres, and 3D
- Citation to related publication:
- Trung, H.-S., Liemohn, M.W. Ilie, R. (2019). Steady State Characteristics of the Terrestrial Geopause [Data set]. University of Michigan Deep Blue Data Repository. https://doi.org/10.7302/7w13-kq27
- Discipline:
- Science
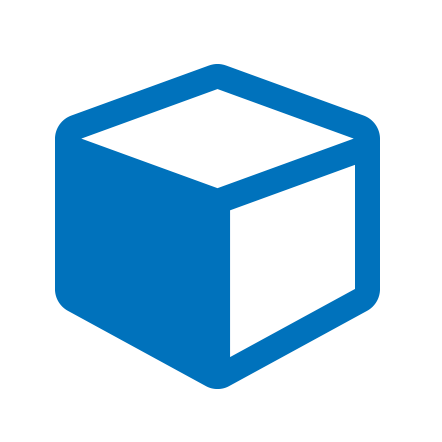
- Creator:
- Liemohn, Michael W
- Description:
- Earth’s upper atmosphere above 500 km altitude constantly loses charged particles to outer space in a process called ionospheric outflow. This outflow is important for the dynamics of the near-Earth space environment (“space weather”) yet is poorly understood on a global scale. A mission is needed to observe the global patterns of ionospheric outflow and its relation to space weather driving conditions. The science objectives of such a mission could include not only the reconstruction of global outflow patterns but also the relation of these patterns to geomagnetic activity and the spatial and temporal nature of outflow composition. A study is presented to show that four well-placed spacecraft would be sufficient for reasonable outflow reconstructions.
- Keyword:
- ionosphere, magnetosphere, satellite mission concept, and space weather
- Citation to related publication:
- Liemohn, M. W., Jörg-Micha Jahn, Raluca Ilie, Natalia Y. Ganushkina, Daniel T. Welling, Heather Elliott, Meghan Burleigh, Kaitlin Doublestein, Stephanie Colon-Rodriguez, Pauline Dredger, & Philip Valek (2024). Reconstruction analysis of global ionospheric outflow patterns. Journal of Geophysical Research Space Physics, 129, e2023JA032238. https://doi/org/10.1029/2024JA032238
- Discipline:
- Science
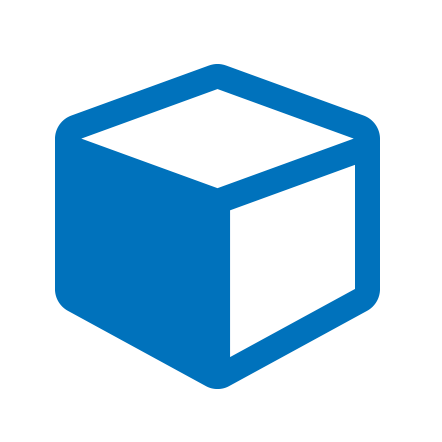
- Creator:
- Trung, Huy-Sinh, Liemohn, Michael W, and Ilie, Raluca
- Description:
- This research is from the last time step of a 12 hour simulation. The files that are attached with this simulation require the raw data from (doi:10.7302/fwq2-ey41). The python files generate various plots for the paper.
- Keyword:
- Space Physics, Magnetospheres, and Magnetohydrodynamics
- Citation to related publication:
- Trung, H.-S., Liemohn, M. W., & Ilie, R. (2019). Steady State Characteristics of the Terrestrial Geopauses. Journal of Geophysical Research: Space Physics, 124(7), 5070–5081. https://doi.org/10.1029/2019JA026636
- Discipline:
- Science
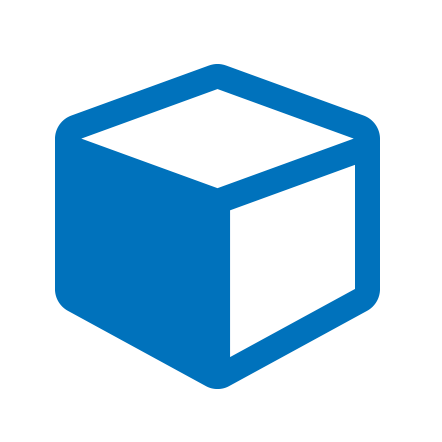
- Creator:
- Liemohn, Michael W, Adam, Joshua G, and Ganushkina, Natalia Y
- Description:
- Many statistical tools have been developed to aid in the assessment of a numerical model’s quality at reproducing observations. Some of these techniques focus on the identification of events within the data set, times when the observed value is beyond some threshold value that defines it as a value of keen interest. An example of this is whether it will rain, in which events are defined as any precipitation above some defined amount. A method called the sliding threshold of observation for numeric evaluation (STONE) curve sweeps the event definition threshold of both the model output and the observations, resulting in the identification of threshold intervals for which the model does well at sorting the observations into events and nonevents. An excellent data-model comparison will have a smooth STONE curve, but the STONE curve can have wiggles and ripples in it. These features reveal clusters when the model systematically overestimates or underestimates the observations. This study establishes the connection between features in the STONE curve and attributes of the data-model relationship. The method is applied to a space weather example.
- Keyword:
- space physics, statistical methods, and STONE curve
- Citation to related publication:
- Liemohn, M. W., Adam, J. G., & Ganushkina, N. Y. (2022). Analysis of features in a sliding threshold of observation for numeric evaluation (STONE) curve. Space Weather, 20, e2022SW003102. https://doi.org/10.1029/2022SW003102
- Discipline:
- Science
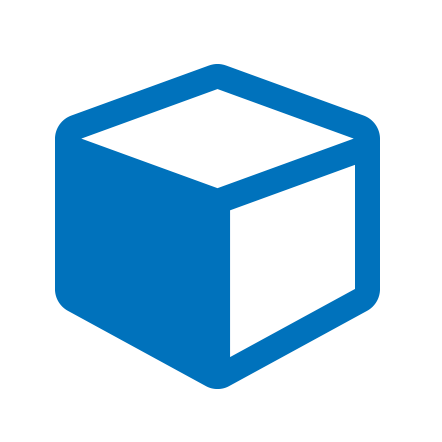
- Creator:
- Liemohn, Michael W
- Description:
- The editorial decision process for the Journal of Geophysics Research Space Physics is assisted by over 1,000 scientists every year, providing over 3,000 reviews per year. These statistics are presented for the years 2013 through 2018, showing some fluctuations but, overall, consistency in the response of the space physics research community to requests to serve as manuscript reviewers. Over half of these reviews are submitted on time, and the average time to review actually dropped as the load increased. This is greatly appreciated and the community is to be commended and thanked for their willingness to help make this journal thrive and remain a premiere publication in the field.
- Keyword:
- Editorial and reviewer statistics
- Citation to related publication:
- Liemohn, M. W. (2020). Editorial: Multiyear analysis of JGR Space Physics reviewing statistics. Journal of Geophysical Research Space Physics, 125, e2019JA027719. https://doi.org/10.1029/2019JA027719
- Discipline:
- Science
- Creator:
- Liemohn, Michael W and Wooden, Paige
- Description:
- Journals sometimes focus the attention of the research community by having a special collection, sometimes an entire special issue, devoted to a single topic. A reasonable question to ask is whether the extra effort of organizing, promoting, and maintaining the special collection is worthwhile. The paper that this data set accompanies examines paper impact in the Journal of Geophysical Research Space Physics, separating the special collection papers from the non-special-collection submissions. The conclusion is that special collections are worth the extra work.
- Keyword:
- journal special collections, bibliometrics, citations, and downloads
- Citation to related publication:
- Liemohn, M. W., & Wooden, P. (2019). Editorial: Impact of special collections in JGR Space Physics. Journal of Geophysical Research Space Physics. https://doi.org/10.1002/essoar.10501036.2
- Discipline:
- Science
- Creator:
- Liemohn, Michael W, Azari, Abigail R, Ganushkina, Natalia Yu, and Rastätter, Lutz
- Description:
- Scientists often try to reproduce observations with a model, helping them explain the observations by adjusting known and controllable features within the model. They then use a large variety of metrics for assessing the ability of a model to reproduce the observations. One such metric is called the relative operating characteristic (ROC) curve, a tool that assesses a model’s ability to predict events within the data. The ROC curve is made by sliding the event-definition threshold in the model output, calculating certain metrics and making a graph of the results. Here, a new model assessment tool is introduced, called the sliding threshold of observation for numeric evaluation (STONE) curve. The STONE curve is created by sliding the event definition threshold not only for the model output but also simultaneously for the data values. This is applicable when the model output is trying to reproduce the exact values of a particular data set. While the ROC curve is still a highly valuable tool for optimizing the prediction of known and pre-classified events, it is argued here that the STONE curve is better for assessing model prediction of a continuous-valued data set. and Data and code were created using IDL, but can also be accessed with the open-source Gnu Data Language (GDL; see https://github.com/gnudatalanguage/gdl)
- Keyword:
- ROC curve, STONE curve, data-model comparison, model validation, forecasting, and statistical methods
- Citation to related publication:
- Liemohn, M. W., Azari, A. R., Ganushkina, N. Yu., & Rastätter, L. (2020). The STONE curve: A ROC-derived model performance assessment tool. Earth and Space Science, 7, e2020EA001106. https://doi.org/10.2019/2020EA001106
- Discipline:
- Science
- Creator:
- Liemohn, Michael W, McCollough, James P, Engel, Miles A, Jordanova, Vania K, and Morley, Steven K
- Description:
- There is a directory tree inside this zipped file. The main directory has the Adobe Illustrator plots of the figures in the paper, Space Weather journal manuscript # 2018SW002067, "Model evaluation guidelines for geomagnetic index predictions" by M. W. Liemohn and coauthors. The three subdirectories have the files for the individual models, the data to which they are compared, and the IDL code used to create the figure plots and metrics calculations. and Date coverage is specific to each model. The RAMSCB model covers January 2005, the WINDMI model all of 2014, and the UPOS model 1.5 solar cycles, from 1 October 2001 through 29 July 2013.
- Keyword:
- space weather, model assessment, time series metrics, and geomagnetic indices
- Citation to related publication:
- Liemohn, M. W., et al. (2018). Model evaluation guidelines for geomagnetic index predictions. Space Weather, 16. https://doi.org/10.1029/2018SW002067
- Discipline:
- Science