Search Constraints
Number of results to display per page
View results as:
Search Results
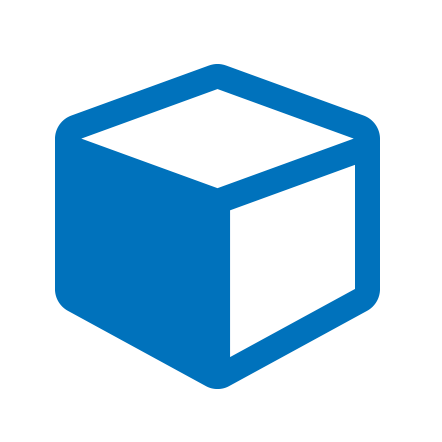
- Creator:
- Ramasubramani, Vyas
- Description:
- The goal of the work is to elucidate the stability of a complex experimentally observed structure of proteins. We found that supercharged GFP molecules spontaneously assemble into a complex 16-mer structure that we term a protomer, and that under the right conditions an even larger assembly is observed. The protomer structure is very well defined, and we performed simulations to try and understand the mechanics underlying its behavior. In particular, we focused on understanding the role of electrostatics in this system and how varying salt concentrations would alter the stability of the structure, with the ultimate goal of predicting the effects of various mutations on the stability of the structure. There are two separate projects included in this repository, but the two are closely linked. One, the candidate_structures folder, contains the atomistic outputs used to generate coarse-grained configurations. The actual coarse-grained simulations are in the rigid_protein folder, which pulls the atomistic coordinates from the other folder. All data is managed by signac and lives in the workspace directories, which contain various folders corresponding to different parameter combinations. The parameters associated with a given folder are stored in the signac_statepoint.json files within each subdirectory. The atomistic data uses experimentally determined protein structures as a starting point; all of these are stored in the ConfigFiles folder. The primary output is the topology files generated from the PDBs by GROMACS; these topologies are then used to parametrize the Monte Carlo simulations. In some cases, atomistic simulations were actually run as well, and the outputs are stored alongside the topology files. In the rigid_protein folder, the ConfigFiles folder contains MSMS, the software used to generate polyhedral representations of proteins from the PDBs in the candidate_structures folder. All of the actual polyhedral structures are also stored in the ConfigFiles folder. The actual simulation trajectories are stored as general simulation data (GSD) files within each subdirectory of the workspace, along with a single .pos file that contains the shape definition of the (nonconvex) polyhedron used to represent a protein. The logged quantities, such as energies and MC move sizes, are stored in .log files. The logic for the simulations in the candidate_structures project is in the Python scripts project.py, operations.py, and scripts/init.py. The rigid_protein folder also includes the notebooks directory, which contains Jupyter notebooks used to perform analyses, as well as the Python scripts used to actually perform the simulations and manage the data space. In particular, the project.py, operations.py and scripts/init.py scripts contain most of the logic associated with the simulations.
- Keyword:
- Protein assembly, Cryo TEM, Hierarchical Assembly, Monte Carlo simulation, and Coarse-grained simulation
- Citation to related publication:
- Anna J Simon, Vyas Ramasubramani, Jens Glaser, Arti Pothukuchy, Jillian Gerberich, Janelle Leggere, Barrett R Morrow, Jimmy Golihar, Cheulhee Jung, Sharon C Glotzer, David W Taylor, Andrew D Ellington,"Supercharging enables organized assembly of synthetic biomolecules," bioRxiv 323261; doi: https://doi.org/10.1101/323261
- Discipline:
- Science and Engineering
- Creator:
- R Paul Drake
- Description:
- The specific focus of the project was radiative shocks, which develop when shock waves become so fast and hot that the radiation from the shocked matter dominates the energy transport. This in turn leads to changes in the shock structure. Radiative shocks are challenging to simulate, as they include phenomena on a range of spatial and temporal scales and involve two types of nonlinear physics Ð- hydrodynamics and radiation transport. Even so, the range of physics involved is narrow enough that one can hope to model all of it with sufficient fidelity to reproduce the data. CRASH was focused on developing predictions for a sequence of experiments performed in Project Year 5, in which those experiments represented an extrapolation from all previously available data. The previous data involved driving radiative shocks within cylindrical structures, and mainly straight tubes. The Year 5 experiments drove a radiative shock down an elliptical tube. Our long-stated goal for these predictions was that the distribution of predicted values would overlap significantly with the observed distribution. We achieved this goal. Achieving our goal required the conversion of an established space-weather code to model radiative shocks at high energy density. To obtain reasonable fidelity with respect to the experimental data required implementing a laser absorption package, in addition to a hydrodynamic solver, electron physics and heat conduction, and multigroup diffusive radiation transport. The dedicated experiments provided evidence of experimental variability, validation of the calculation of initial shock wave behavior, and validation data at many observation times using cylindrical shock tubes. Following this were preparatory experiments for and finally the execution of the Year 5 experiments. The predictive science research included a wide range of sensitivity studies to determine which variables were important and a sequence of predictive studies focused on specific issues and sets of data. This led ultimately to predictions of shock location for the Year 5 experiments. A conclusion from this project is that the serious quantification of uncertainty in simulations is a dauntingly difficult and expensive prospect. Pre-existing codes are unlikely to have been built with attention to what will be needed to quantify their uncertainty. Pre-existing experimental results are even more unlikely to include a sufficiently detailed analysis of the experimental uncertainties. And this will also be true of most experiments that might be used to validate components of the simulation. The analysis of uncertainty in any one of the physical processes (and related physical constants) is a major effort. And addressing model form uncertainty is an even bigger challenge, that may in principle require development of complete, alternative simulation models. We made a start at all of this, and completed almost none of it. But by the end of a project, we finally had all the pieces in place and working that would have enabled a range of important studies and advances in relatively near-term years. But the sponsor terminated the program after only five years. For most of the participants this was a relatively minor development, although for a few of them it proved to be enormously disruptive. We believe that the cost to the nation, in work that was ready be done but now will not be, was much much larger. The sketch of the target was produced using a drawing program based on the experimental dimensions. The annotated photograph of the target was obtained using a visible-light camera. The colorized radiographs were obtained via backilit-pinhole radiography of a radiative shock propagating down an elliptical tube, at 26 ns after the lasers driving the shock tube fired. The graph showing lines and circles was produced by running many computer models, analyzing their statistical distribution, and measuring actual shock positions in the experiment.
- Keyword:
- Radiative shock
- Citation to related publication:
- R.P. Drake, F.W. Doss, R.G. McClarren, M.L. Adams, N. Amato, D.Bingham, C.C. Chou, C. DiStefano, K. Fidkowsky, B. Fryxell, T.I.Gombosi, M.J. Grosskopf, J.P. Holloway, B. van der Holst, C.M.Huntington, S. Karni, C.M. Krauland, C.C. Kuranz, E. Larsen, B. vanLeer, B. Mallick, D. Marion, W. Martin, J.E. Morel, E.S. Myra, V. Nair, K.G. Powell, L. Raushberger, P. Roe, E. Rutter, I.V. Sokolov, Q. Stout, B.R. Torralva, G. Toth, K. Thornton, A.J. Visco, “Radiative Effects in Radiative Shocks in Shock Tubes”, High Energy Density Physics 7, 130-140 (2011) https://doi.org/10.1016/j.hedp.2011.03.005
- Discipline:
- Science
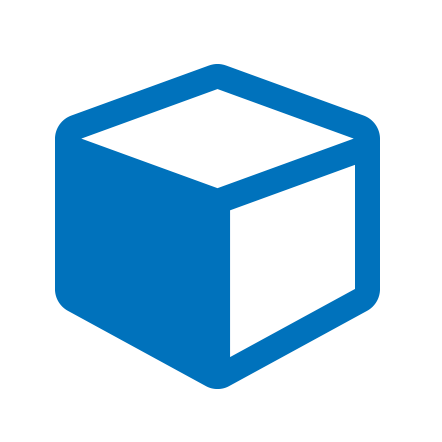
- Creator:
- Adams, Julia
- Description:
- This eportfolio was created for the Gateway course of the Sweetland Minor in Writing to provide an opportunity for students to reflect on their growing identities as writers, as captured in their text-based and multimodal compositions produced over the Gateway semester. The title of the work contains the pseudonym created for the study while the creator field lists the student's given name to allow proper attribution for their work. The eportfolio is collected here as an artifact in the Sweetland Writing Development Study, which has been published as Developing Writers in Higher Education: A Longitudinal Study (University of Michigan Press, 2019). To learn more about this study, please see the epublication https://doi.org/10.3998/mpub.10079890, and to learn more about the Minor in Writing program and the eportfolio prompts, please see Appendix 2a - https://doi.org/10.3998/mpub.10079890.cmp.1 to the publication.
- Keyword:
- eportfolio , gateway, sitesucker, website, and file directory
- Citation to related publication:
- Gere, A.R., Editor. Developing Writers in Higher Education: A Longitudinal Study, fulcrum.org. University of Michigan Press. https://doi.org/10.3998/mpub.10079890
- Discipline:
- Humanities
- Creator:
- Liemohn, Michael W, McCollough, James P, Engel, Miles A, Jordanova, Vania K, and Morley, Steven K
- Description:
- There is a directory tree inside this zipped file. The main directory has the Adobe Illustrator plots of the figures in the paper, Space Weather journal manuscript # 2018SW002067, "Model evaluation guidelines for geomagnetic index predictions" by M. W. Liemohn and coauthors. The three subdirectories have the files for the individual models, the data to which they are compared, and the IDL code used to create the figure plots and metrics calculations. and Date coverage is specific to each model. The RAMSCB model covers January 2005, the WINDMI model all of 2014, and the UPOS model 1.5 solar cycles, from 1 October 2001 through 29 July 2013.
- Keyword:
- space weather, model assessment, time series metrics, and geomagnetic indices
- Citation to related publication:
- Liemohn, M. W., et al. (2018). Model evaluation guidelines for geomagnetic index predictions. Space Weather, 16. https://doi.org/10.1029/2018SW002067
- Discipline:
- Science
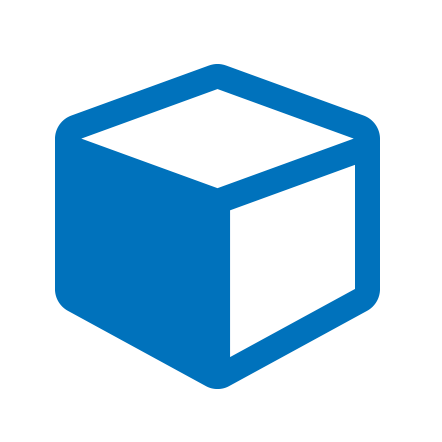
- Creator:
- Kort, EA, Gvakharia, A, Smith, ML, and Conley, S
- Description:
- Data is collected from research flights based in West Memphis, Arkansas, covering the Mississippi River Valley. The data file contains all merged flight data from each flight day.
- Keyword:
- Greenhouse gas
- Citation to related publication:
- Gvakharia, A., Kort, E.A., Smith, M.L., Conley, S., 2018. Testing and evaluation of a new airborne system for continuous N2O, CO2, CO, and H2O measurements: the Frequent Calibration High-performance Airborne Observation System (FCHAOS). Atmospheric Measurement Techniques; Katlenburg-Lindau 11, 6059. https://doi.org/10.5194/amt-11-6059-2018
- Discipline:
- Science
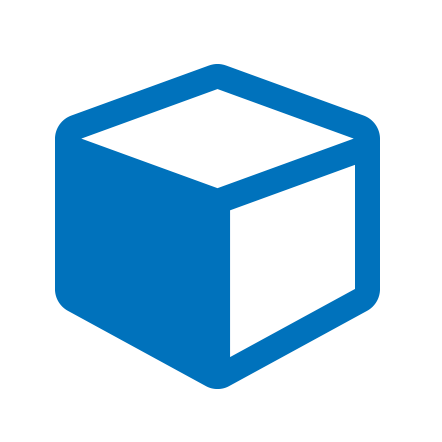
- Creator:
- Lee, Kyu Han, Foxman, Betsy, and Gordon, Aubree
- Description:
- Data include variables used to run mixed effects models examining the association between the nose/throat microbiome and influenza virus infection. Certain individual participant data have been excluded due to identifiability concerns. Data also include the oligotype count table and taxonomic classifications. and Curation Notes: Readme updated Nov. 29, 2018 with context for oligotype and taxonomy files, and citation to associated article.
- Keyword:
- Influenza and Microbiome
- Citation to related publication:
- Lee KH, Gordon A, Shedden K, Kuan G, Ng S, Balmaseda A, Foxman B. The respiratory microbiome and susceptibility to influenza virus infection. PloS One. 2019;14:e0207898. https://doi.org/10.1371/journal.pone.0207898
- Discipline:
- Health Sciences
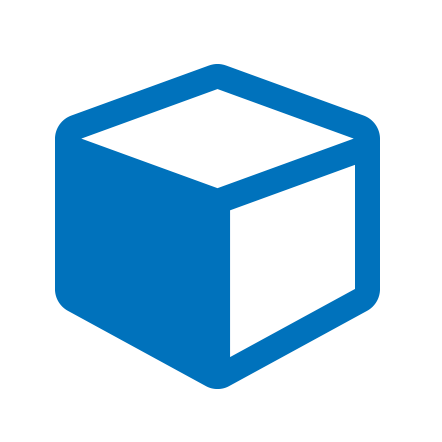
- Creator:
- Reed, Matthew P., Boyle, K.
- Description:
- This is the first physical anthropomorphic test device to be based both on statistical body shape models as well as 3D printing.
- Keyword:
- Child Belt Fit Manikin
- Citation to related publication:
- Reed, M.P. and Boyle, K.J. (2017). Development of a manikin representing a two‐year‐old child for belt‐fit measurement. Proc. 2017 IRCOBI Conference. Antwerp, Belgium. https://trid.trb.org/view/1486130 and Kim, K.H., Jones, M.L.H., Ebert, S.M., Malik, L., Manary, M.A., Reed, M.P., and Klinich, K.D. (2015). Development of Virtual Toddler Fit Models for Child Safety Restraint Design. Technical Report UMTRI-2015-38. University of Michigan Transportation Research Institute, Ann Arbor, MI.
- Discipline:
- Engineering
- Creator:
- Isaacoff, Benjamin P., Li, Yilai, Lee, Stephen A., and Biteen, Julie S.
- Description:
- This is the experimental data referenced in our manuscript entitled “SMALL-LABS: An algorithm for measuring single molecule intensity and position in the presence of obscuring backgrounds .” These live-cell single-molecule imaging movies were used as a test of the SMALL-LABS single-molecule image analysis algorithm. The dataset comprises two movies; each one is provided both as a .tif stack and as an .avi file. The movie called “low_bg” has a standard low background, and the movie called “high_bg” includes a high fluorescent background produced by an external 488-nm laser.
- Keyword:
- single-molecule, microscopy, image analysis, mirobiology, and bacteria
- Citation to related publication:
- B.P. Isaacoff, Y. Li, S.A. Lee, J.S. Biteen, "SMALL-LABS: Measuring Single-Molecule Intensity and Position in Obscuring Backgrounds." Biophysical Journal, 975-982, 116, 2019. https://doi.org/10.1016/j.bpj.2019.02.006
- Discipline:
- Engineering and Science
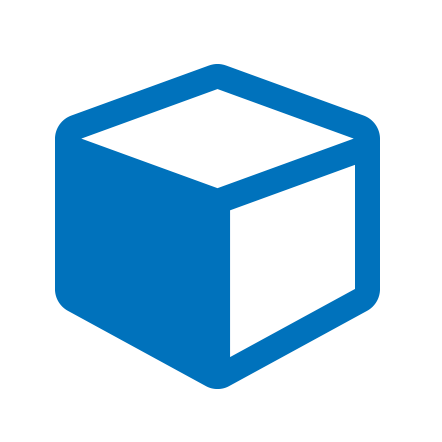
- Creator:
- Thomaz, Andréa T. (UMICH) and Knowles, L. Lacey (UMICH)
- Description:
- The eastern coastal basins of Brazil are a series of small and isolated rivers that drain directly into the Atlantic Ocean. During the Pleistocene, sea-level retreat caused by glaciations exposed the continental shelf, resulting in enlarged paleodrainages that connected rivers that are isolated today. Using Geographic Information System (GIS), we infer the distribution of these paleodrainages, and their properties for the east Brazilian coast. Here, we publicly make available the shapefiles that demonstrate the paleodrainage structure along the Brazilian coast during the largest sea-level retreats in the Pleistocene, the riverine vectors during the same period and the coastal line for a drop of -125m in the sea.
- Keyword:
- Paleodrainages, Glaciations, Pleistocene, Brazil, Neotropical, and Sea-level retreat
- Citation to related publication:
- Thomaz Andréa T., Knowles L. Lacey. Flowing into the unknown: inferred paleodrainages for studying the ichthyofauna of Brazilian coastal rivers. Neotrop. ichthyol. [Internet]. 2018; 16(3): e180019. http://dx.doi.org/10.1590/1982-0224-20180019
- Discipline:
- Science
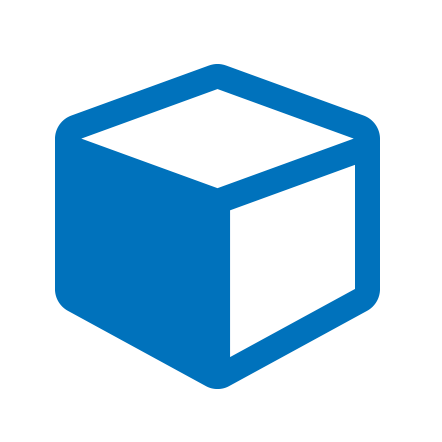
- Creator:
- Alsip, Peter
- Description:
- Percent Weight Change Data: The model was run continuously on a daily time step for seasonal intervals (Spring: March thru May; Summer: June thru August; Fall: September thru November) as well as contiguously from Spring to Fall to assess total growth over the likely growing season (March thru November). CSV files represent the simulated weight change (%) of Bighead and Silver Carp for the respective time periods associated with the file name. Initial fish mass for each seasonal interval and growing season was 4350 g for Silver Carp and 5480 g for Bighead Carp. Maximum and mean total weight change (%) was determined for three depth ranges (near surface depths [NS]: 0 – 10 m; deep chlorophyll layer depths [DCL]: 10 - 50 m; and whole water column [WC]). Coordinates are in decimal degrees. File naming convention: speciesSeasonWtChange (e.g. bigheadFallWtChange = % weight change of Bighead Carp from September through November) , Monthly Habitat Quality Data: Rdata files contain matrices of Bighead or Silver carp growth rate potential as represented as a mass-proportional growth rate (gram of carp/gram of carp/day [g/g/d]) for the 15th day of each month. Habitats with growth rate potential >= 0 g/g/d were deemed suitable. Matrix attributes: Rows: Row numbers refer to the spatial node with 20 equally-spaced vertical layers. Columns: Columns 1-20 refer to the growth rate potential value for each vertical layer of each node. Vertical layers are evenly spaced based on the total depth of the water column for each node. Depth for each node can be found in the grid attributes data file. Columns 21 ("meanG") and 22 ("Gmax") represent the average and maximum growth rate potential, respectively, of the fish across the whole water column for the corresponding node. File naming convention: species_MonthNumber (e.g. silver_06 = Silver carp growth rate potential in June) Spatial coordinates for each node can be found in the grid attributes data files., Grid attributes data: This Rdata file provides the spatial reference data and other grid attributes. Coordinates are provided in UTM (x & y) and latitude and longitude (decimal degrees). Depth (meters) for each node is listed in this file. , GRP Model code: Details bioenergetics equations, foraging equation, functions for running the model on a monthly time-step and daily time step, and functions for basic analyses. Model is coded in R., and The simulated input data (prey and temperature) used to run our model is not included in this data set. Instead we provide the model code, grid attributes, and outputs of the model. The readRDS() function (R Base Package v.3.5.1) is required to read in .Rdata files in R.
- Keyword:
- Asian Carp, Laurentian Great Lakes, Habitat Suitability, Invasive Species, Lake Michigan, and Ecological Modeling
- Discipline:
- Science